A massive quantity of data is needed for log analysis. Entrepreneurs can accumulate a few megabytes per day, whereas the biggest network organizations, such as Facebook, record terabytes of data every day.
It’s Pointless to Try to Analyze Logs Manually
The majority of logs are uninteresting and of no benefit. It is not possible to individually read from each line of log info. You're looking for a fork in a haystack, in other words. You look for terms like "bug," "fired," and "abort" in your quest. You might discover an electrical gremlin in this direction whether you're fortunate, but you'll almost certainly skip main issues that are not found in the findings of the keyword you checked for.
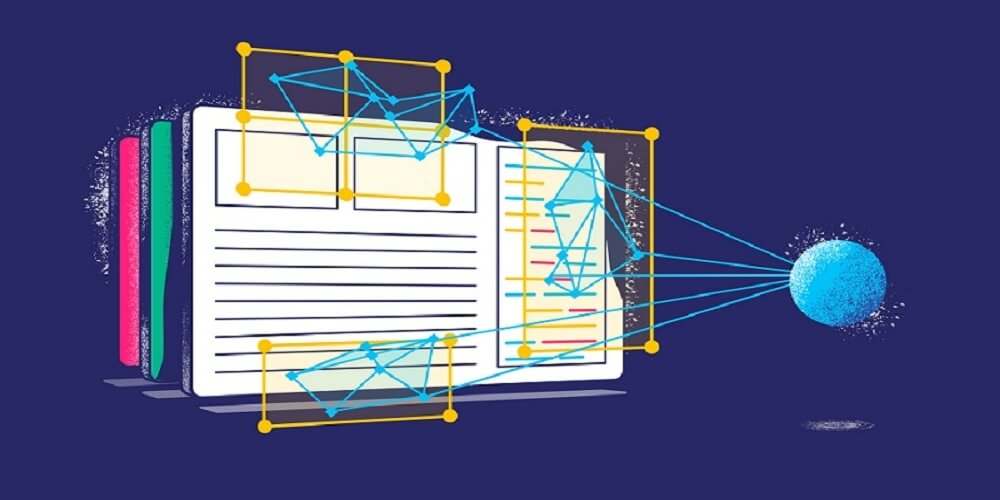
The knowledge of the individual performing software testing services is essential. They might achieve little traction manually checking logs if they do have a thorough knowledge of the issue, are aware of present changes, and are aware of how each component of the system functions regularly. Nevertheless, for a DevOps unit, this is a significant disadvantage. It places the team's fate in the hands of a single individual, but if that individual is not accessible or unable to fix the problem, the whole activity is jeopardized.
In a production or quality assurance system, a manual log review can be possible. It is, nevertheless, uncontrollable in output. Path to confidential log data is limited in development, and administrators are unable to search log data by jumping from one server to another one in a wide area.
Machine Learning Log Analysis is herein providing the Solution
Machine learning may be a portion of, if not the whole, and the answer to conventional log analysis problems. The ability of machines to defeat mankind has been shown. This capability enables machines to drive vehicles, recognize photographs, and identify cyber-attacks in projects that involve a big quantity of data.
Tech teams will eliminate repetitive and reproducible activities with artificial having to learn log review, freeing up engineers to concentrate on processes that computers can't handle. Real concern projects and brainstorming about potential products are two examples.
Machine Learning for Log Analysis Functionalities
Using machine learning in combination with log picture analysis helps you to categorize data quickly. Since logs are textual data, NLP techniques may be used to arrange the same logs in an ordered way, allowing you to look for unique log forms. One of the advantages of machine learning is that it identifies issues and concerns immediately, even though there is a substantial percentage of a log.
Certain intrusion detection systems generate needless notifications that are, in most situations, not the source of actual problems. It is possible to be notified when anything has to be addressed through machine learning. This eliminates the problem of positive result warnings. There is often an original phenomenon that's not observed with certain catastrophic cases. This phenomenon could be detected using machine learning until it becomes a big issue.
1. Coralogix of the highest quality
Coralogix adheres to the strictest privacy and protection requirements offering several territorial data centers to comply with different local privacy laws. Genuine timings, SaaS monitoring, analytics, and SIEM enables teams to satisfy the demands of digital technology in terms of size, efficiency, and expense.
2. The Datadog
Datadog is a log analysis application that uses a SaaS-type analytics visualization tool to provide tracking of applications, systems, devices, and facilities. Datadog's analysis shows log data as diagrams, allowing you to see how network success has changed over time. Datadog utilizes centralized storage space and artificial intelligence to spot unusual log trends and problems, as well as centralized data management to prevent log data from ever being corrupted.
3. Loggly / SolarWinds
This is a packed, inter-log processing system that is expensive, centralized, and modular. It combines accurate detection and optimization with robust notifying, dashboarding, and monitoring to proactively detect issues and dramatically minimize Long Run to remedy. Rapidly scan through vast and dynamic systems to see integrated systems, incidents, and problems, processing large amounts of information.
4. Logz.io
Logz.io is built on cloud-native technology, making it simple to connect with your remote applications, databases, K8s networks, virtualized features, and the majority of the applications. Logz.io is system data analytics software based on Grafana that is knowledgeable and versatile. Logz.io is a cloud-native management framework that integrates publicly available machine learning with virtualized functionality and extensibility to enable engineers to detect potential problems before they happen and enable them to track, fix problems, and protect mission-critical apps using a single cohesive framework.
5. Splunk
Computer data has grown at an unprecedented rate over the last period, and you should be mindful of this. It was partially due to the expanded usage of connected systems and partly due to the number of computers in the IT network. This computer data contains a wealth of useful knowledge that can help the company increase performance, competitiveness, and exposure. Splunk was created in 2003 with a single goal in mind: to make sense of computer log data, and the market for Splunk expertise has grown steadily ever since. It is a well-known commercial log integrating tool.
Final Thoughts
Numerous log analysis systems engage machine learning services to aid in the automated identification of underlying problems and disputes, eliminating the need for extensive or systematic analysis. Do you want to waste time building your log analysis method from scratch, or would you rather have a platform that does it for you so you can concentrate on your company? Value the time you will save by selecting a stream processing program, in addition to the functionalities and expenditure.