This blog offers a gentleman’s perspective to imbalanced classification. It also introduces diverse approaches to re-calibrate the process with different techniques.
Examples to Study Predictive Modeling
Just to explain imbalance classification, a few examples are mentioned below. They will help you to understand and develop a case study for a new predictive modeling. A real world example of electricity theft has already been discussed throughout this content. The other areas where such problems occur are in financial and large volumes of biometric data. In sensitive areas of security applications, military and defense related algorithms it needs better handling.
Snapshot of common domains where problems occur during predictive modeling
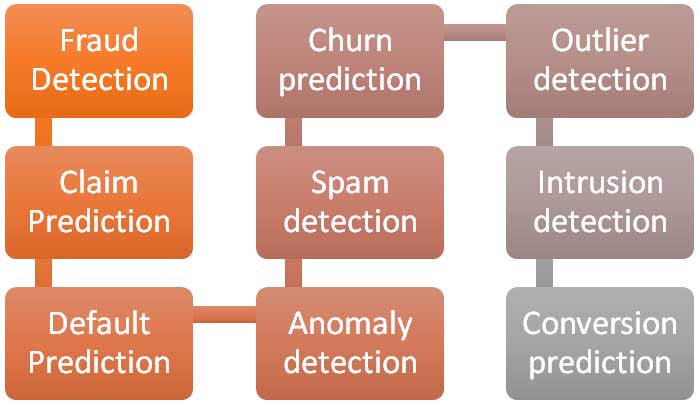
Each area by itself can be explored for imbalance classification through multiple issues. Only a very skilled and experienced professional can understand the challenges associated with it.
Index of Priority Keynotes
- Overcoming challenges related to data samples
- Imbalance classification causes
- Probable approach to handling the samplings
- Examples to study for predictive modeling
Overcoming Challenges Related to Data Samples
The incorporation of artificial intelligence solutions in the computer programs, helps to make more well-organized and actual systems. The chance in the method of AI is thought-provoking and well-organized at the same time.
The obvious pitfall to be kept in mind while talking about the competences and the prospects offered by this space-age world is that the quantity of information being created on a day-to-day basis is quickly increasing and it is being impossible to pit and examine the information fully. The amount of data generation has made it difficult for the individuals to deal with. It has surpassed the competences of persons that they can mine the valued info out of it.
The capable professionals in the area of data science with the proficiency and their ability sets try to make correlations among numerous inputs so that they could draw out a precise output. However with the absolute capacity of data, it has become comparatively not possible to associate each possible input. Thus, Artificial Intelligence can help in Integrating AI into the systems to purify the rare facts into palatable and useful information.
Artificial intelligence is even handled by the innovative and fresh codes normally mentioned as algorithms. AI can make existing applications easier to use, more intuitive to user behavior and more aware of changes in the environment they run in
In many industrial areas, electricity theft is a problem. Many enterprises are looking at addressing this challenge by developing predictive models via machine learning.
Advanced analytics are used to understand the patterns of energy consumption to detect thefts. As unstructured data is collected across distribution channels, it is difficult to process the same. For example, can healthy transactions be segregated from fraudulent ones who steal electricity?
With machine learning consulting services In India, it is not very easy to produce classifications and it leads to imbalance and inaccuracy. The main trial lies in getting the right data sampling to measure imbalance probabilities.
Even regular evaluations done with decision tree and logistics regression fail to produce truthful results. This makes one to return to the origin of imbalance classification triggers which could be slight or severe in nature depending on algorithms used.
Imbalance Classification Causes
As mentioned earlier, the two main causes for imbalance account for inaccuracy include data sampling and property domains. There are other minor issues also, however these two major areas need more attention for problem solving. There is a strong possibility of wrong measurement of the data sample and the way it has been collected.
The other reflections for errors include:
- Conservative geographical location from where samples are gathered.
- The time range is wrong. The samples should have been amassed from different time zones for better reading.
- Different methods have been used to get data sets.
- During analysis, errors are overlooked.
- Sometimes the processes to collect are damaged and alternative systems bring up new results that do not match.
- Often the imbalance is linked to property domain.
- One class may dominate the others creating a clear disparity.
- Cost factors and low resources reduce accuracy of results.
- A wrong predictive model is used to collect data.
These details lead us to ask if there is a systematic approach that could be utilized right from the start of the trails. There are few ways that are mentioned below.
Probable approach to Handling the samplings
The problem can be approached from the data samples, use of random under and over sampling. To get better results cluster-based oversampling is beneficial. The experts have to use strategies to improve the algorithms and balance the classes even before the pre-processing period. This ensures there is a wider application of the data set.
When a balance is achieved, the minority and majority class frequency is in sync. This is done via resampling the collection of information. When under sampling is done the majority class is eliminated on a random basis.
So, if the main objective is to closely monitor the electricity usage, the non-fraudulent cases and combined with the fraudulent ones. This could benefit the storage but however, it could be biased. It may not be able to give an accurate representation.
The same happens when an over sampling method is involved. One major advantage with this technique is that no data is lost. The cluster-based technique is independent and is applied to minor and major classes separately. This way the dataset clusters are identified. In fact, this method is most useful to overcome the challenges posed by imbalance classifications.
Importance of knowledge to an increasing business
When the info is used appropriately the depiction lets AI methods to work with almost human intelligence. The growing use of usual language even creates it like humans in its responses.
Final thoughts:
There is no one stop solution for dealing with imbalance classification. The techniques will continue to evolve as real world challenges come up. The user will have to depend on the characteristics of the dataset to determine which solution could be applicable to get a new predictive model.
Using previous model comparisons is also useful. We have more articles related to this and to look out for that just check below Read More links. Thank You!